As someone investing in dozens of channels – social media, search advertising, email, blogging and more – you want to know which are giving the best return on investment. You want a reliable multi-channel attribution analysis that lets you:
- Better allocate your marketing and advertising budget
- Get reliable data about the effect that marketing activities have on conversion rates
- Decrease the cost of acquisition per marketing channel
But how can you do that when the customer journey is so fragmented? Going from first touch to conversion rarely happens within one browsing session. The process spans across many channels and touchpoints, and doesn’t happen overnight. One more thing: precise attribution requires collecting personal data. The appearance of increasingly strict regulations such as CCPA and GDPR make this a delicate operation.
Add all those elements together and you get this: multi-channel attribution analyses are harder than ever. And they were never easy to begin with.
Many organizations make the problem worse by relying on a last-click attribution model. This gives all credit for a conversion to the last step in the journey – any other touchpoints are assumed to have contributed nothing at all. Shaky analyses lead to uneven results. In the end, many throw up their hands in despair and don’t even try to analyze attribution.
The good news is that multi-attribution reports are useful. They aren’t magic reports that will immediately tell you what your highest performing channels are. The reports will give you plenty of indications over time, though, if you know how to design smart experiments around them. This is the approach that analytics experts such as Avinash Kaushik recommend.
To do this, you first need a platform that allows for a flexible, multi-model approach. You’ll also need to collect personal data in a compliant way, especially if you want to track conversions for individual visitors, customers or users.
To start, we’re going to define all the important terms. Then we’ll show you a practical example of how such an analysis could be done in Piwik PRO.
What is multi-channel conversion attribution?
Multi-channel conversion attribution is the process of assigning credit for conversions in a customer journey that spans more than one channel. It’s also sometimes referred to as multi-touch attribution. Some also just talk about attribution, advertising attribution or marketing attribution and assume the multi-channel nature of the analysis.
The question of how to evaluate the effects of different marketing activities goes back at least a century. Claude C. Hopkins wrote about testing and comparing campaigns in 1923, in his classic book, Scientific Advertising. Even if the approach isn’t new, you’d be right in saying that a lot has changed. Measuring campaigns across digital channels is much different than comparing the effects of billboards to those of coupons in newspapers.
The modern process of modeling attribution needs to include more channels. Coupons and billboards may still be relevant for some, but every organization also needs to deal with digital channels. That’s why we prefer the term multi-channel conversion attribution. It puts the focus on just how many channels we now have to think about.
Let’s start by looking at the challenges this multitude of channels presents.
Multi-channel conversion attribution and its challenges
Some treat conversion attribution as a magic solution to all their problems while others claim it doesn’t work at all or isn’t worth the trouble. Analytics expert Avinash Kaushik, among others, offers a reasonable middle ground – lower your expectations and do lots of experiments to compare the results of different approaches.
He also explains that there are different kinds of multi-channel attribution – a fact you need to account for in any attribution analysis project.
- Across digital channels – What most of us have in mind when we think of multi-channel attribution – a single person on a single device moving through touchpoints on different online channels
- Across multiple devices or browsers – Still digital, but now a single identifier, such as a browser cookie, won’t be enough to patch together the customer journey
- Online to store – Tracking the influence of online marketing efforts on offline sales
Want to learn more about tracking across devices? We recommend Clearcode’s post: What is cross-device attribution and what’s so hard about it?
There are even other kinds of attribution for those with more offline campaigns. For example, some businesses want to know the effect that outdoor advertising and paper coupons have on online sales.
The implication is clear, though: Know from the start what kind of attribution you’re working with. Avinash Kaushik points out that many vendors and consultants promise to mix all the types of attribution into one magic analysis, complete with accurate conversion data. It’s a nice dream, but it’s also rarely possible to pull off in practice. Keep that in mind while planning what analyses you want to perform and what kind of results you expect.
To analyze any kind of attribution, we need to know what attribution models are available to us. So that’s what we’ll look at next.
The types of conversion attribution models and how they work
First-click models, also called first-interaction, assign 100% of a conversion to the first known click, traffic source or referrer. Let’s say a visitor enters your website from Google Ads, looks around and then leaves. Later, they come back via an organic Google search link and fill out a form – the conversion. That last visit via organic search won’t be counted at all. The first interaction, via Google Ads, gets 100% of the credit for the conversion.
Last-click models, or last-interaction, give 100% of the credit to the last known click, traffic source or referrer. For the example above, a Google Ad click and then an organic search click, all the credit would go to organic search.
Last-non-direct-click models remove direct visits from the equation. It attributes 100% of conversion to the last known indirect click, traffic source or referral. This is a variation on the last-click model. In Piwik PRO, you would create it with a custom model based on a last-click template. We’ll get into all these customization options in the next section.
Linear models treat each touchpoint equally. The success is attributed in equal measure to each referrer and visit taking place before the conversion. So three touchpoints, say Google Ads, Google organic search, and a direct visit, before a conversion would see each touchpoint getting ⅓ of the credit.
Position-based models attribute X% of credit to the first touch, Y% to the last touch and Z% spread evenly across all the touchpoints in between. This is an easily customizable model, just change the percent inputs, that also gives some credit to all touchpoints.
Time-decay models assign credit to each touchpoint, but the closer the touchpoint is to a final transaction or reaching the goal, the more credit it gets. You set the “half life” and the model calculates the rest. The half life represents the amount of time needed for credit to halve. So for a half life of 7 days, a click 7 days before a conversion would get half the credit of a click the day of conversion.
Marketing expert Lennart Ruigrok, notes that many marketers make the mistake of relying only on the last-click model, which is the default model in Google Analytics. It’s not that the model is bad, but it can cause you to ignore the contribution of touchpoints that may play a significant role in conversion. This is a weakness of the all-or-nothing models – first-click, last-click and last-non-direct-click.
Read the details of how marketing expert Lennart Ruigrok approaches the difficult topic of conversion attribution in affiliate marketing: How to analyze multi-channel attribution in affiliate marketing using raw data.
The strength of the other three models – linear, position-based and time-decay – is that they assign credit to more than one touchpoint. This doesn’t mean they are better for modeling a given conversion funnel, but they are worth experimenting with to spread credit beyond a single touchpoint.
In addition to the standard models, we also need to look at custom models and other advanced features that will improve the quality of our analyses.
Advanced multi-channel attribution features worth using
Custom models
The standard models will take you far, but having a little extra control can help in all sorts of situations. Custom models allow you to modify parts of the standard models to give you that extra control.
For example, say there is a rarely seen touchpoint that is important enough to be given much more credit than all the others, no matter where it is in the path to conversion. The standard models don’t make it possible to single out such touchpoints and give them more credit. So let’s look at the options custom models give us.
The first modifications to consider are the model parameters. Each model has different parameters that can be adjusted.
You could consider parameter changes as variations of standard models or as new custom models. In Piwik PRO, we use the label custom model for any model that has been changed, even a slight change in one parameter, from the standard models.
With all-or-nothing models like first- and last-click, you can set conditions for that one touchpoint that will get all the credit. In this way, not just any first or last touch will get the credit, but only one that meets certain conditions. For example, you could ignore all blog posts and only give credit to sales or landing pages. Or you could limit credit to only external ad campaigns.
The situation looks different for the models that assign credit to multiple touchpoints. With a time-decay model, the most important parameter is the half life, which changes dramatically how credit is distributed across longer customer journeys. With a position-based model, you can adjust the split between first, middle and last interactions. And finally, with a linear model, there aren’t any key parameters to adjust. In Piwik PRO you can also link credit to user engagement, according to session page views or session time, for all three of these models.
The second kind of modifications are additional credit rules.
These additional rules let you add a credit multiplier based on whatever conditions you define. So let’s go back to the example we started this section with, a rarely seen but important touchpoint. Let’s say that our research shows that visitors who go through a product tour are much more likely to move to the next step of the sales process. We want to give this touchpoint 10 times the credit of any others in the customer journey. We would do so with an additional credit rule, as seen in the Piwik PRO screenshot below.
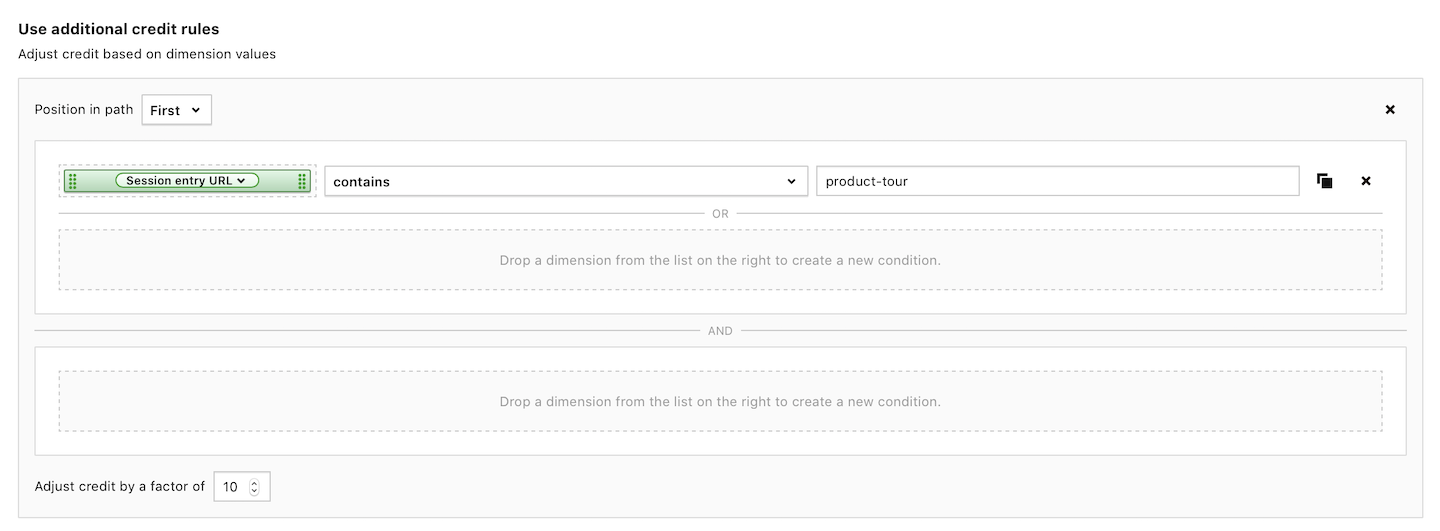
The other parameters of the model would still apply. So in a time-decay model, this touchpoint would get assigned credit based on when it appeared in the customer journey. That credit value would then be multiplied by 10 because of the additional credit rule. The same would be true for linear and position-based models. The base model assigns a value that is then modified by any additional credit rules that apply.
In Piwik PRO, these additional rules also can be used for first-click and last-click models, but they have a slightly different effect. If you have put a filter on which first- or last-click you want to get credit, then additional rules serve as backup in the case that the first filter excludes all touchpoints in a particular conversion path. In this way, the additional rules behave more like secondary filters for all-or-nothing models.
Attribution model attributes and customization options in Piwik PRO
Credit for more than one touchpoint | Adjustable Parameters | Other options | |
---|---|---|---|
First-click | filters | ||
Last-click | filters | ||
Linear | user engagement | additional rules | |
Position-based | credit splits, user engagement | additional rules | |
Time-decay | half life, user engagement | additional rules |
Read more about the technical details of setting up a custom model in our help center article.
Attribution model comparison in Piwik PRO
Now that you have standard and custom models to choose, it might seem like there is too much. Where to start? How to choose the right model?
Comparing models against each other is key. It’s also important to put those comparisons in the context of an experiment with a specific goal. The comparison part is easy:
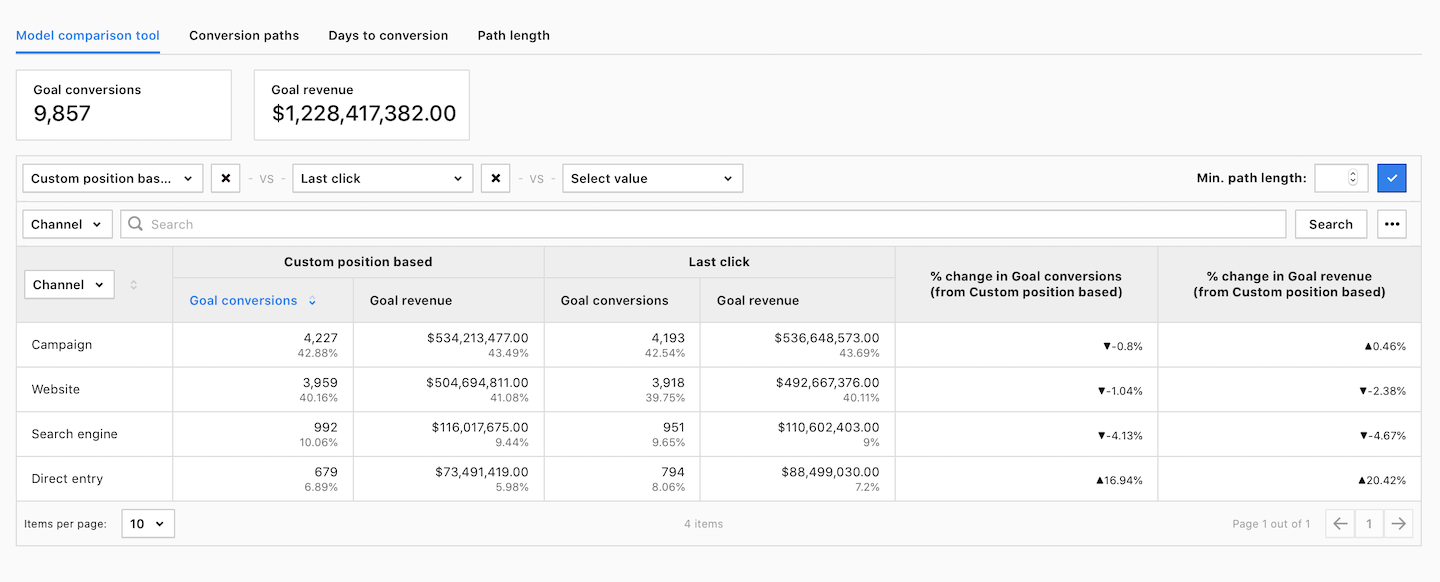
In Piwik PRO, you can quickly compare standard and custom models in any multi-channel attribution report.
Getting the context right can be harder. The questions we’re asking are hard ones. Which multi-channel attribution model is giving me the correct results? Which pages, ads, campaigns, etc. had the most influence in creating this conversion?
To slowly work towards these answers, we need to conduct lots of experiments. Let’s say that one model shows that one Google Ads campaign got the most credit for one kind of conversion. Another model shows that one category of blog posts got the most credit. An experiment could be to do more or less of the Google Ads campaigns or the blog posts and see over time how that affects conversions.
To be clear, this can be a long process. You need to do your best to isolate variables and test the inputs into any conversion – one at a time. Unfortunately, even experienced analytics experts such as Avinash Kaushik, who we discussed in an earlier section, have found that there is no faster way to get reliable answers to questions about attribution.
Read more about applying various attribution models in the Clearcode article: 7 multi-touch attribution models for conversion-driven marketers and the Piwik PRO help center article.
Custom channel grouping
Custom channel grouping lets you treat several traffic sources like a single channel for the purposes of attribution analysis.
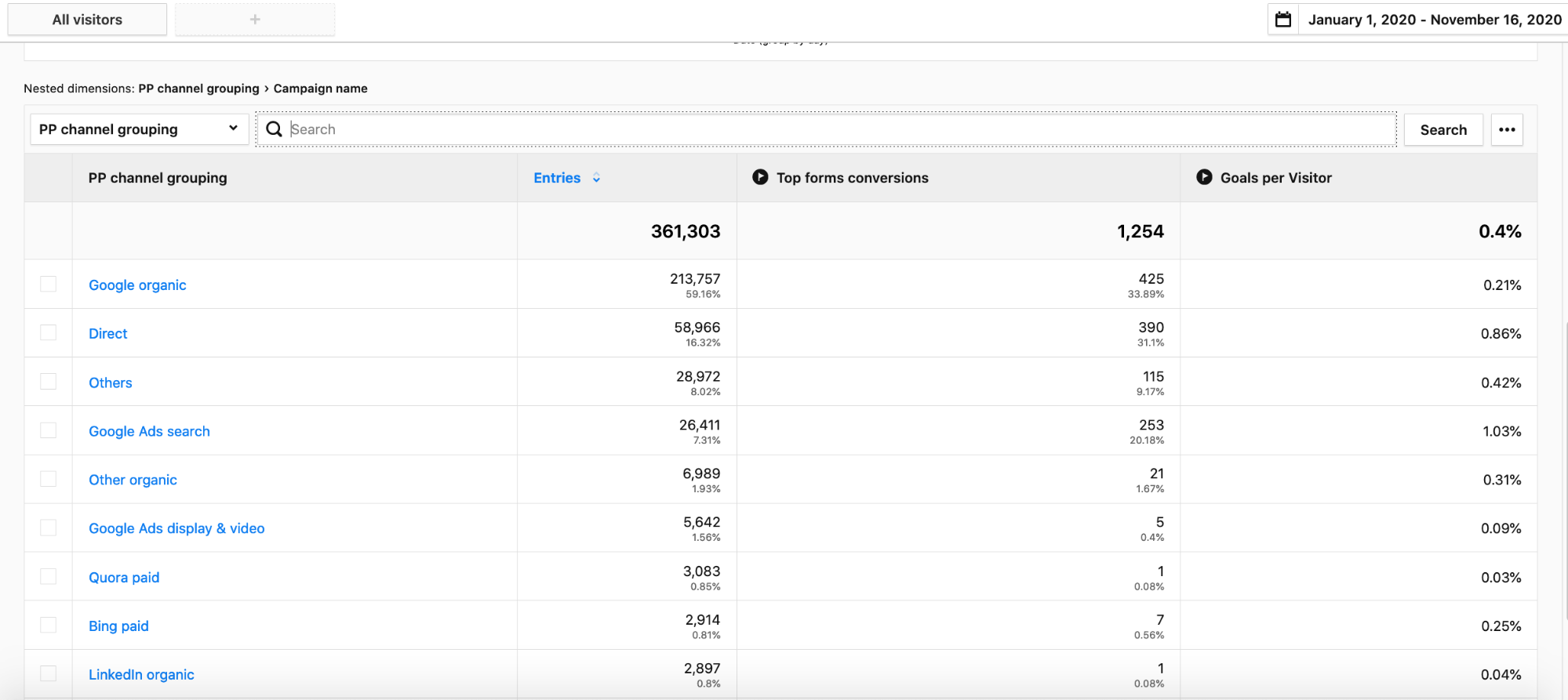
Take for example an analysis of paid versus organic traffic. You could put all paid sources in one channel group and all organic sources in another. You could do this manually, but by treating many channels as one, you can save time and make your reports easier to interpret.
API and raw data access
Built-in reports and custom report builders are great. But sometimes there are certain analyses that require something none of those reports can deliver. Maybe it’s a different way of filtering or presenting the data. Or maybe it involves combining data from another source. In any case, this is where access to a robust API and unlimited raw data is priceless.
Export data en masse for external analyses. Create custom integrations to other platforms that will automatically pull analytics data as needed. The options are endless when you have full access to, and control of, your analytics data.
A built-in consent manager
Many wouldn’t include a built-in consent manager as a part of an attribution toolbox, and yet it’s a crucial component. It will also only get more important as data privacy laws get tighter around the world.
To maximize data collection and the accuracy of your reports, you need a consent manager. Without one, you won’t be able to collect any personal data in many countries. There are many stand-alone options on the market. While they do a decent job, nothing is better than a consent manager that is designed to work specifically on the analytics platform you’re using. You won’t have to worry about losing data due to problems with a complex integration process.
A hands-on example of a multi-channel attribution analysis
We now have a full conversion attribution toolbox. It might even be a little too full. To make it clearer how these tools work together, let’s go through an example.
Let’s say we work at a bank and want to better understand what marketing channels are most efficient at sending us traffic that converts into clients who take out a certain kind of loan.
To start any attribution analysis, we need a hypothesis. Then we need one or more models that we’ll use to accept or reject that hypothesis.
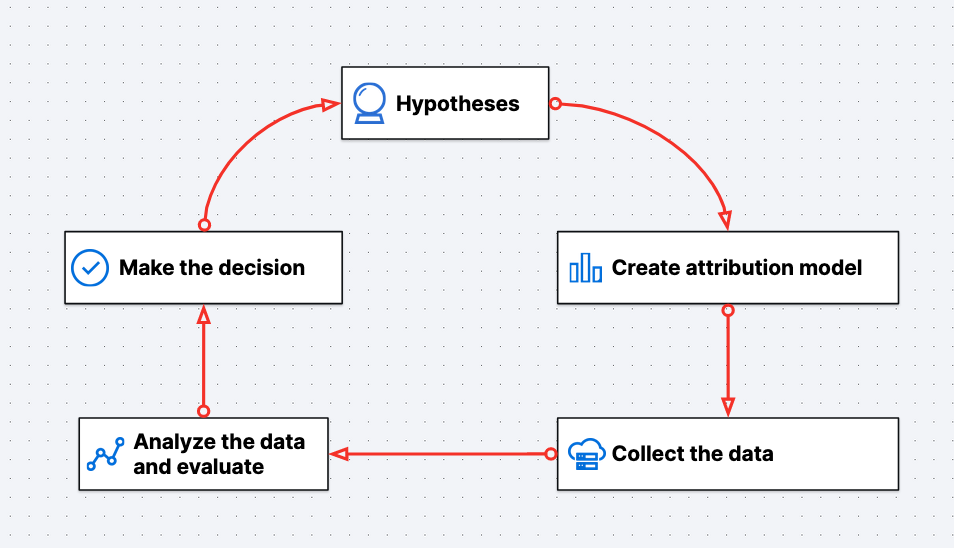
We also need to decide on what time period we’ll look at and which channels we’ll take into account.
For this example, we’ll set up a group of channels, “PP Bank Marketing Channels”, and look at one full month. Our hypothesis is that two affiliate marketing campaigns are working well and are worth continuing.
It’s also worth noting that this analysis will definitely require personal data. Since we’re working with banking information, the data could even be considered sensitive. Having a good way to get consent before collecting any of that data is a crucial part of setting up the analysis.
If you’d like to get more information about analytics in banking and consent requirements, visit our blog:
We can already start to dig into conversions a little bit by looking at days to conversion and path length reports.
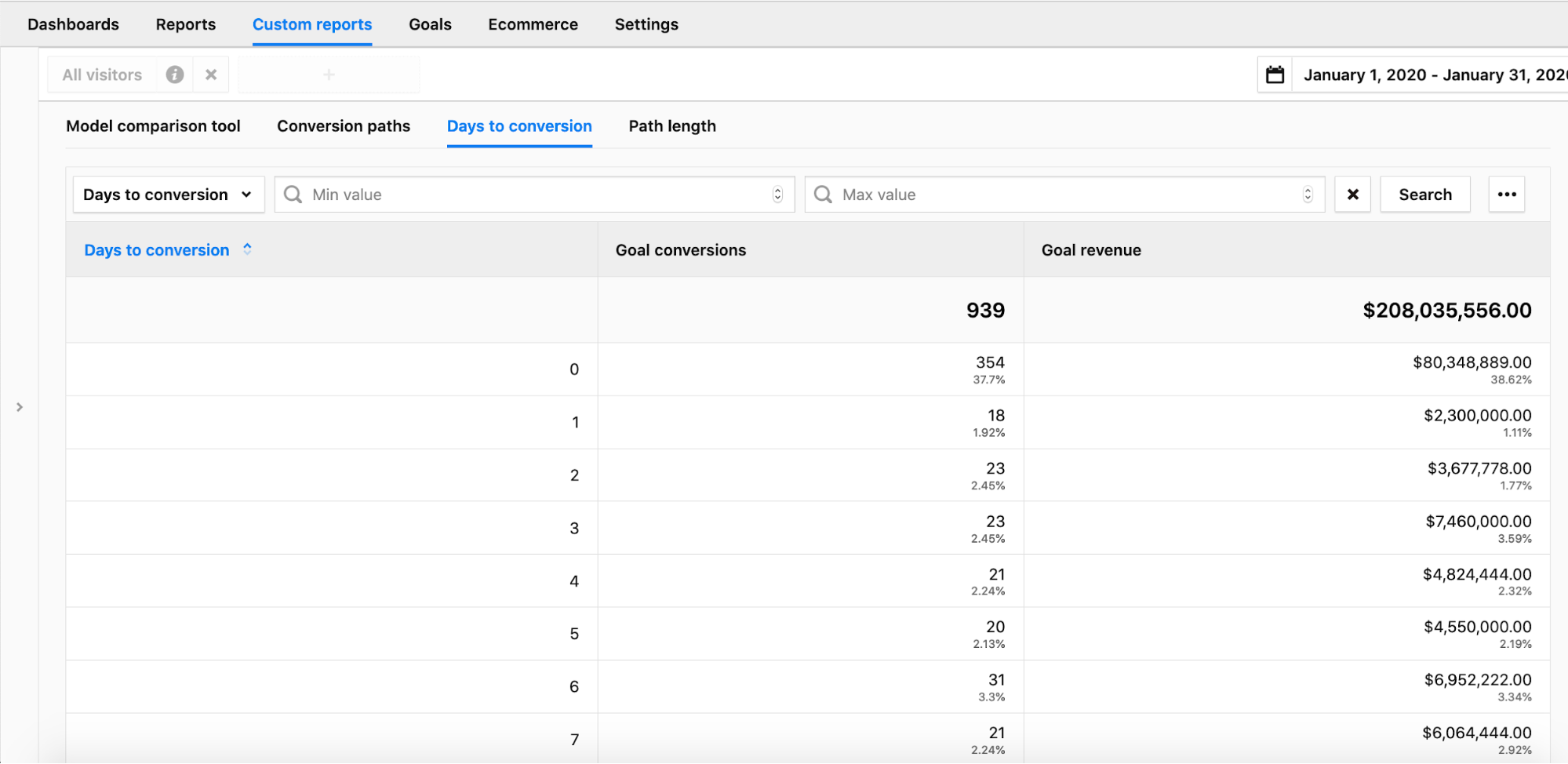
We can also look at a conversion path report to see what touchpoints visitors interact with along the way and in what sequence.
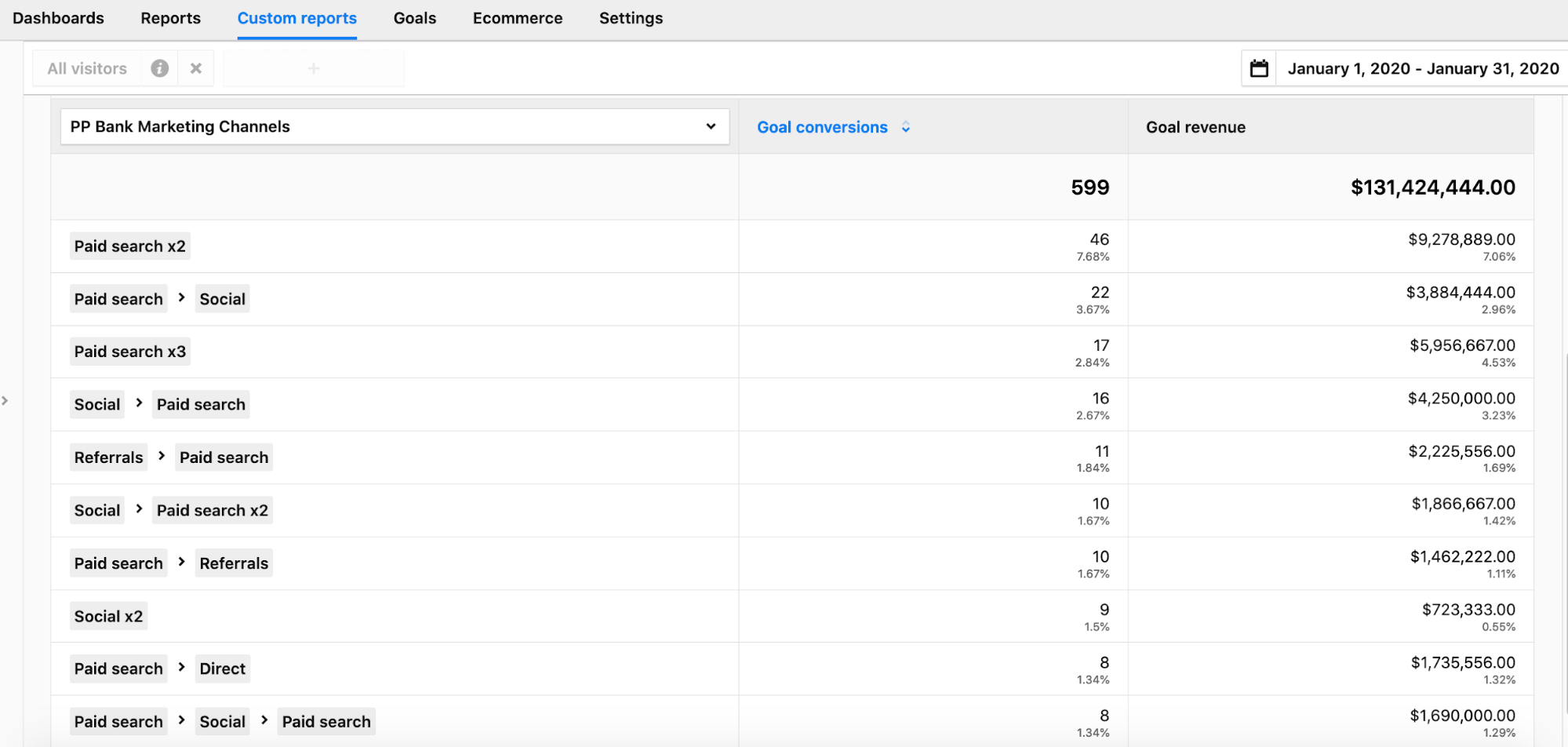
These summary reports are good to get a feel for the customer journey. Eventually though, we need to start creating models that will assign credit to each channel. This will allow us to prove or disprove the hypothesis that our two affiliate campaigns are working.
We’ll use three models to check: a last-click model, a first-click model and custom position-based model. In the custom model, we’ll give more, but not all, credit to the first interaction.
Then we can take a look at the number and value of conversions produced by each channel according to the models.
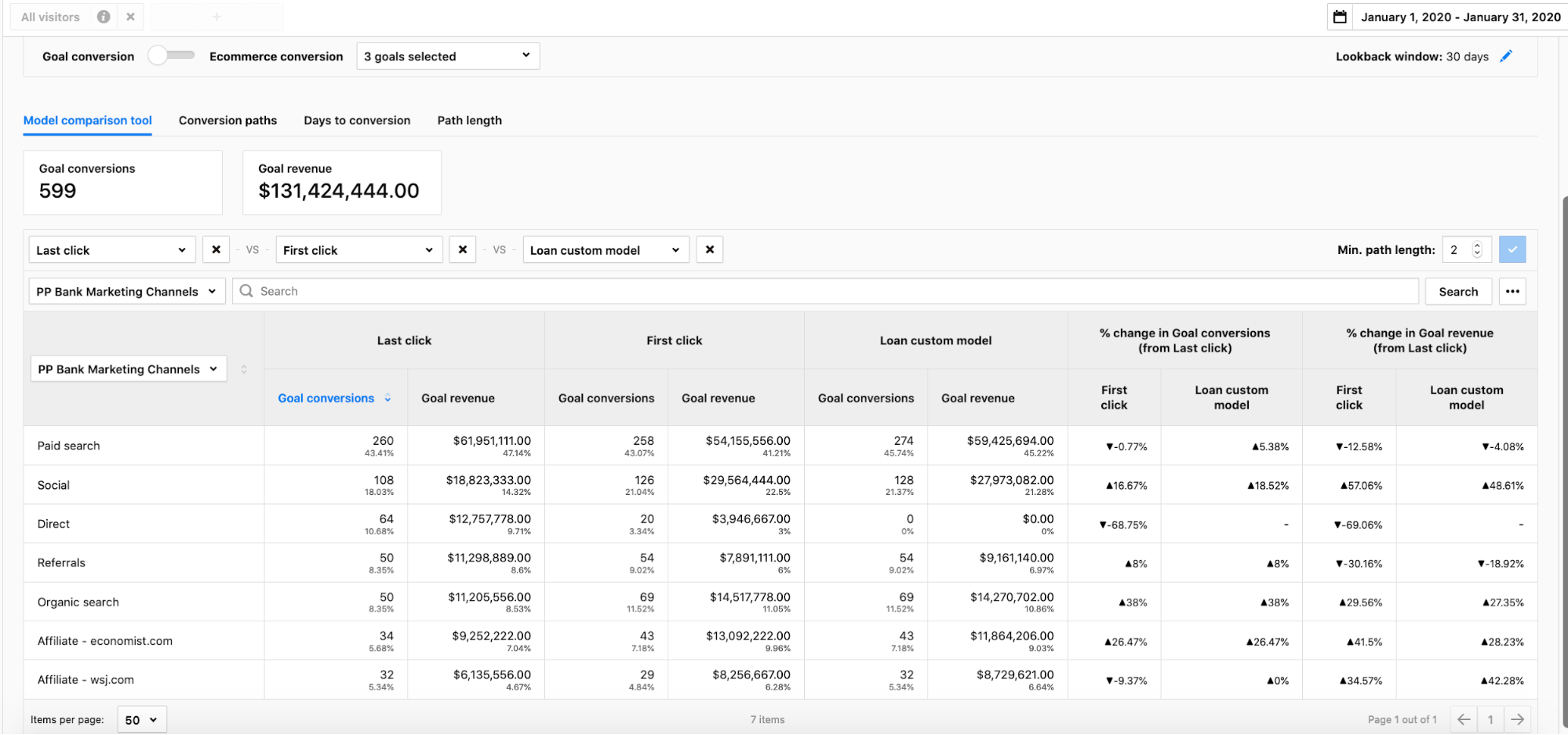
We can now check the costs for running those affiliate campaigns and see if they have positive ROI and are worth continuing. But that wouldn’t be the end of our analysis. It would be just the start.
Now we have a performance baseline. We can track metrics, including these attribution reports, over time and see what has positive and negative effects. We could also take an active approach and make changes based on our own research or on market trends. We can then monitor the effects and compare back to that baseline we established.
For example, we could double the spending on a paid campaign and see if we get twice the revenue. That’s likely not to be the case, so we’d have to check ROI like we did for the affiliate campaigns.
Use multi-channel conversion attribution to “Be less wrong over time”
As Avinash Kaushik explains well, all attribution models have their pros and cons. So it’s not about picking the perfect model the first time. In his words, success comes “from your ability to take that rough output, make changes, observe the impact (over weeks, or months if you are small-sized), identify insights and be less wrong over time.”
In a way, this should come as a relief. You don’t have to get it 100% right the first time! What’s more, you’ll probably never be 100% right, at least not when it comes to conversion attribution.
We hope this article has encouraged you to try out (or come back to) multi-channel attribution analyses.
Read other related articles follow the links:
>> How funnel reports can improve conversion rates and user experience
(opens in a new tab)”>>>> How funnel reports can improve conversion rates and user experience
>> Why first-party data is the most valuable to marketers
(opens in a new tab)”>>>> Why first-party data is the most valuable to marketers